Optimizing ALM models: Loan prepayments and turbulent times
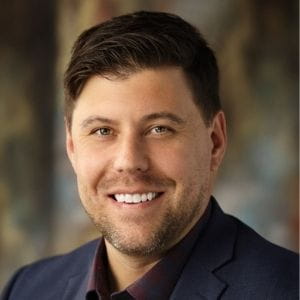
Amid the changing landscape for financial institutions, it’s essential to stay on top of the factors affecting asset and liability management (ALM) model inputs, including loan prepayment speeds.
Prepayments play a pivotal role in shaping ALM model results, and as interest rates fluctuate, so do the cash flows from your loan portfolio. Mortgage loans serve as a prime example of how prepayment speeds can fluctuate. During periods of rising interest rates, consumers often hesitate to refinance or move due to higher potential payments, resulting in slower loan prepayments. This trend extends the duration of your loan portfolio.
On the other hand, in a declining rate environment, loan prepayments accelerate as more individuals opt for refinancing, leading to a shorter duration for the loan portfolio.
To effectively manage interest rate risk, your institution must make informed decisions about your loan prepayment assumptions. Model managers can draw on a variety of sources, including:
- National averages: Public sources like Fannie Mae or Freddie Mac provide data on prepayments, which can serve as a benchmark.
- Data vendors: Third-party data vendors offer prepayment calculators that consider factors like collateral type, incentive to refinance, collateral age and burnout, among others.
- Historical data: You can leverage your institution’s past data to determine prepayment speeds, potentially isolating different rate environments and collateral types.
- A blend of approaches: Some institutions combine their historical data with third-party or national averages.
Use these approaches in conjunction with qualitative adjustments. Consider specific characteristics such as local market competition, institution-specific strategies or the presence of prepayment penalties. For instance, institutions that do subprime consumer lending should expect different prepayment behaviors compared to A-credit loans.
Market dynamics can also influence prepayment speeds, as heightened competition can incentivize refinance activity. If using historical data, also consider the time frame when the data was captured, and whether those particular behaviors apply to today’s interest rate environment.
To understand risks embedded in your ALM model results, it’s crucial to run annual sensitivity tests for loan prepayments. These tests help you understand the directional risk associated with prepayment speeds. We recommend modeling slower prepayments in rising rate scenarios and faster prepayment speeds in falling rate environments.
During times of volatility, relying solely on historical data may not be sufficient. National averages and data vendors also often overlook the unique credit qualities, markets and collateral characteristics of your institution’s loan portfolio. Depending on your loan portfolio, it may be important to assess your source for loan prepayments and make qualitative adjustments to align them with your institution’s distinct features.
How Wipfli can help
By reviewing the data, understanding the factors that set your institution apart and incorporating these adjustments into your ALM model, you can gain confidence in your strategies and help ensure that your institution remains successful. Wipfli’s ALM consultants can help you feel secure in your ALM model management. We can provide a trusted ALM validation and a comprehensive overview of your ALM process.
Contact us to learn more about our ALM services.
Sign up to receive additional financial institutions content in your inbox or continue reading on: