How your organization can make use of your data with AI in financial services
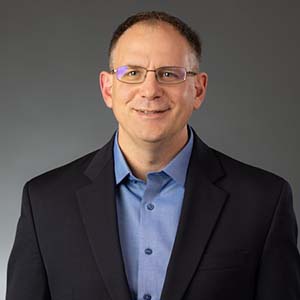
Resilient organizations don’t stop looking for ways to improve, even amid challenges. They’re always trying innovative solutions that can help them be competitive and continue to grow.
For financial services organizations that want to stay resilient, data may be one of your greatest untapped assets.
Some organizations are already taking advantage of the insights data and analytics can provide. But you can build on those capabilities further by incorporating artificial intelligence (AI) and machine learning. With AI in financial services, your organization can optimize performance and make smarter business decisions.
Here are some areas where your organization can use AI and machine learning to stay ahead:
Forecasting
AI and machine learning can help you make smarter business decisions with forecasting.
Today’s financial institutions aren’t just dealing with cash flow from loans and deposits. They also need to manage things such as investment fees, insurance premiums and fees from third-party vendors. Machine learning helps you forecast incorporating these noninterest, income-related aspects of cash flow so that you can make more effective decisions around revenue.
You can also use machine learning for other important aspects of your business, such as lending, accounts payable or accounts receivable.
By analyzing customer behavior data, machine learning can identify the characteristics of customers who may be struggling with their payments, allowing you to be proactive in monitoring potential delinquent borrowers. You can quickly assess their status and, if necessary, reach out to them and adjust their terms.
Implementing forecasting often requires collaboration that stems from planning. Forecasting solutions typically include collaborative features to accept input from multiple team members or departments, audit logs and handle version management. These features strengthen the forecasts while offering additional data for the machine learning models.
Fraud detection
Using AI and machine learning helps ensure your organization’s fraud detection reflects the latest changes and fraud behaviors.
Many organizations still rely on rule-based fraud detection, which uses rigid, set conditions to test transactions. If your organization isn’t keeping up with current fraud behaviors, or if the fraud falls outside the boundaries of those conditions, the tests fail.
With machine learning, you can build statistical models that can identify outliers to normal conditions and probabilities of incidents that may be fraud, helping you find conditions that you didn’t anticipate. You can also easily keep models fresh through routine training and testing.
Automation
Automation helps your organization’s people spend less time managing data and more time using it for strategic work.
It can be difficult to take advantage of data in financial services because of the sheer amount an organization can generate. Even for something as simple as transactions, it would be impossible for an individual to sort through historical data to find the transactions relevant to the insights they want.
This data also tends to be isolated.
Many times, organizational processes are disconnected because of disparate systems. These disconnected systems then affect critical cashflow based processes such as accounts receivable and accounts payable.
For example, you may have different delinquencies recorded in separate systems. To know the total delinquency, you’d have to run separate reports and then compile that data manually.
AI and machine learning combined with process integrations can do all that work for you. It helps you bring data together, enabling you to see it all in one place. And this simple increase in visibility through modern data visualizations over your processes can expose information required for better decision making.
Marketing
Machine learning helps you maximize your marketing efforts by using data from customer profiles and behaviors.
At a population level, machine learning can identify the characteristics of customers who are fully banked or who you’re having the most success with. This helps you narrow down the demographics that your organization should be targeting.
On an individual level, statistical analysis, or models such as next-best action, can identify what services a customer may want based on what similar customers use, enabling you to make personalized cross-selling offers. These models also help increase customer awareness of your organization’s services.
How Wipfli can help
At Wipfli, our consultants have the knowledge and experience you need to maximize your data. We can help you at any stage of your data transformation journey, whether you’re focused on data engineering or the development of machine learning models.
Contact us today to learn more about how we can help support your organization.
This article is part of our series on how your financial services organization can use technology to build resilience. We cover the latest technologies and strategies to improve efficiency, engage customers and increase revenue in any economic conditions.
Sign up to receive more financial services industry content in your inbox or continue reading from our series:
- How iPaaS integrates with legacy banking systems for a digital tomorrow
- The importance of understanding risk and resilience
- Benefits of building resilience in the workplace
- Digital transformation for financial services
- Overcome challenges with CRM in financial services
- Strategies for customer retention in financial services
- Four investments to recession-proof your business